
What is Data Governance
“Data Governance is the practice of efficiently and effectively managing the availability, usability, integrity and security of data assets in an organisation.”
How to approach the design of a Data Governance Framework
What is the difference between a theory-based approach and an ontology-based approach to defining a Data Governance framework?
At WiRD Associates we have use two primary approaches to designing a data governance framework, these are a theory-based approach and an ontology-based approach.
- Ontology-based data governance uses a formal representation of the domain of interest, called an ontology, to define the data governance rules and policies. This approach is particularly useful for managing complex data sets, such as big data, where there is a lot of heterogeneity and ambiguity.
- Theory-based data governance uses a theoretical framework to define the data governance rules and policies. This approach is more flexible than ontology-based data governance, but it can be more difficult to implement.
The differences between these two approaches are summarised below.
Feature |
Ontology-based data governance |
Theory-based data governance |
Formal representation |
Uses an ontology to represent the domain of interest |
Uses a theoretical framework to represent the domain of interest |
Flexibility |
Less flexible |
More flexible |
Ease of implementation |
More difficult to implement |
Easier to implement |
Suitability for complex data sets |
More suitable for complex data sets |
Less suitable for complex data sets |
Feature |
Ontology-based data governance |
Theory-based data governance |
The optimal approach to any data governance will depend on the specific needs of the organisation.
If the organisation has complex data sets that need to be managed effectively, then ontology-based data governance may be the best option.
However, if the organisation needs a more flexible approach, then theory-based data governance may be a better choice.
At WiRD Associates we have found the following additional criteria should be considered when choosing between ontology-based data governance and theory-based data governance:
- The size and complexity of the organization’s data sets
- The level of expertise of the organization’s data governance team, i.e., maturity
- The budget available for data governance
- The regulatory requirements that the organisation needs to comply with
Ultimately, the best way to choose between ontology-based data governance and theory-based data governance is to consult with an experienced data governance professional.
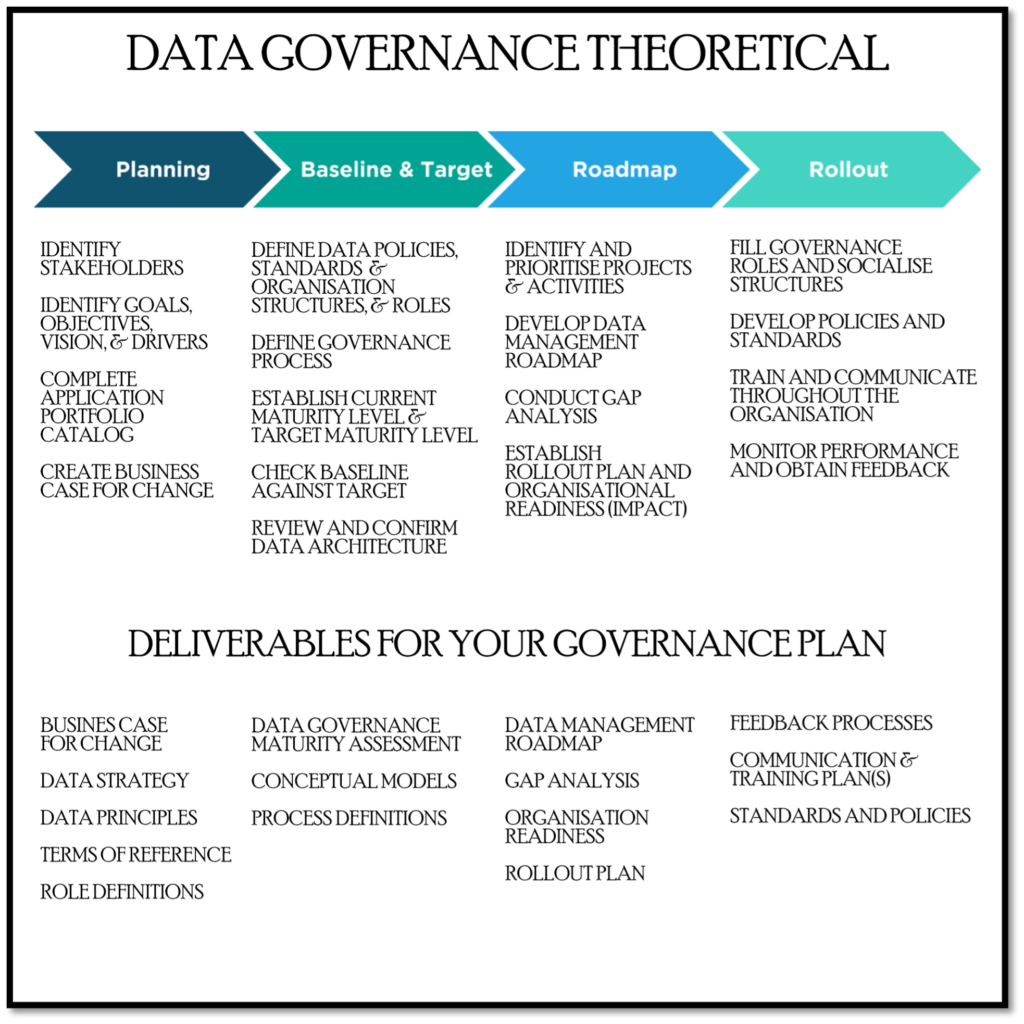
An example of a conceptual Theory based data governance model.
The conceptual Theory based data model is based on the theory of stakeholder management. This theory states that organisations should identify and manage the interests of all of their stakeholders, including employees, customers, suppliers, and regulators.
The model is divided into three primary phases:
- Phase 1: Identification of stakeholders. This phase involves identifying all of the stakeholders who have an interest in the organisation’s data.
- Phase 2: Assessment of stakeholder needs. This phase involves assessing the needs of each stakeholder in terms of data governance.
- Phase 3: Development of data governance policies and procedures. This phase involves developing policies and procedures that are designed to meet the needs of all of the stakeholders.
The model is flexible and can be adapted to the specific needs of the organisation. It can be used to develop a comprehensive data governance framework that is aligned with the organisation’s business goals and objectives.
Here are some of the key concepts that are used in the model:
- Stakeholder: A stakeholder is anyone who has an interest in the organization’s data.
- Data governance: Data governance is the process of managing the data assets of an organisation in a way that meets the needs of all of its stakeholders.
- Policy: A policy is a rule or guideline that is used to govern the behaviour of individuals or organisations.
- Procedure: A procedure is a step-by-step guide that is used to implement a policy.
The model can be used to develop policies and procedures for a variety of data governance activities, such as:
- Data ownership: This policy defines who owns the data in the organization.
- Data access: This policy defines who has access to the data and what they can do with it.
- Data retention: This policy defines how long data should be kept.
- Data security: This policy defines the measures that are taken to protect data from unauthorised access, use, disclosure, disruption, modification, or destruction.
- Data quality: This policy defines the standards that are used to measure the quality of data.
The model can also be used to develop policies and procedures for specific data governance initiatives, such as:
- Data integration: This initiative involves combining data from different sources into a single, consistent view.
- Data mining: This initiative involves using statistical techniques to extract patterns and trends from data.
- Data visualization: This initiative involves using graphical techniques to represent data in a way that is easy to understand.
This is just a simple example of a conceptual theory-based Data Governance model. The specific details of the model will vary depending on the specific needs of your organisation.
WiRD Associates have found that the conceptual Ontology Data Governance model is best divided into three main layers:
- The conceptual layer: This layer defines the core concepts of data governance, such as data, metadata, data quality, and data security. In our view this layer is the foundation of the model. It provides a common understanding of the concepts of data governance, which is essential for effective communication and collaboration.
- The governance layer: This layer defines the policies and procedures for managing data, such as data ownership, data access, and data retention. In our experience this layer defines the policies and procedures that are used to manage data. These policies and procedures should be aligned with the organisation’s business goals and objectives.
- The technology layer: This layer defines the technologies that are used to implement data governance, such as data warehouses, data lakes, and data quality tools. In our experience this layer provides the tools and infrastructure that are needed to implement data governance. These tools should be selected based on the specific needs of your organisation.

An example of a conceptual Ontology based data governance model.
Key Concepts that are defined in the conceptual layer of the Ontology data governance model.
- Data: Data is any information that is collected and stored by an organization.
- Metadata: Metadata is data that describes other data. It can be used to understand the meaning of data, how it is used, and how it is related to other data.
- Data quality: Data quality is the degree to which data meets the needs of its users. High-quality data is accurate, complete, consistent, and timely.
- Data security: Data security is the protection of data from unauthorized access, use, disclosure, disruption, modification, or destruction.
The governance layer of the model defines the following policies and procedures:
- Data ownership: This policy defines who owns the data in the organisation.
- Data access: This policy defines who has access to the data and what they can do with it.
- Data retention: This policy defines how long data should be kept.
- Data security: This policy defines the measures that are taken to protect data from unauthorised access, use, disclosure, disruption, modification, or destruction.
The technology layer of the model includes the following technologies:
- Data warehouses: Data warehouses are used to store large amounts of data for analysis.
- Data lakes: Data lakes are used to store all of an organisation’s data, regardless of its format or structure.
- Data quality tools: Data quality tools are used to identify and correct errors in data.
This is just a simple example of a conceptual Ontologies-based Data Governance model. The specific details of the model will vary depending on the specific needs of the organisation.
In summary, WiRD has found that formal data governance is important in today’s business place for a number of reasons, including:
- To improve data quality. Data governance helps to ensure that data is accurate, complete, and consistent. This is essential for making informed decisions, detecting fraud, and complying with regulations.
- To reduce risk. Data governance helps to mitigate the risks associated with data, such as data breaches, data loss, and data misuse.
- To increase efficiency. Data governance can help to streamline data processes, improve collaboration, and reduce costs.
- To comply with regulations. Many industries are subject to data regulations, such as the General Data Protection Regulation (GDPR) and the Consumer Privacy Act (CPA). Data governance can help organisations to comply with these regulations and avoid fines.
- To make better decisions. Data-driven decision-making is becoming increasingly important in today’s business world. Data governance helps to ensure that organisations have access to the right data, at the right time, in the right format, so that they can make better decisions.
Key to an optimal data governance framework is selecting the right approach to designing the framework, a theory-based approach, or an Ontology based approach.
What is your experience in this regard? How has your organisation approached formal Data Governance and what has the pros and cons been of that approach? Please share your experiences with WiRD and each other by commenting or interacting on LinkedIn
this post was written in collaboration with Poena le Roux and Tommy Steyn