- 2023-08-23
- Posted by: admin
- Category: IT Architecture
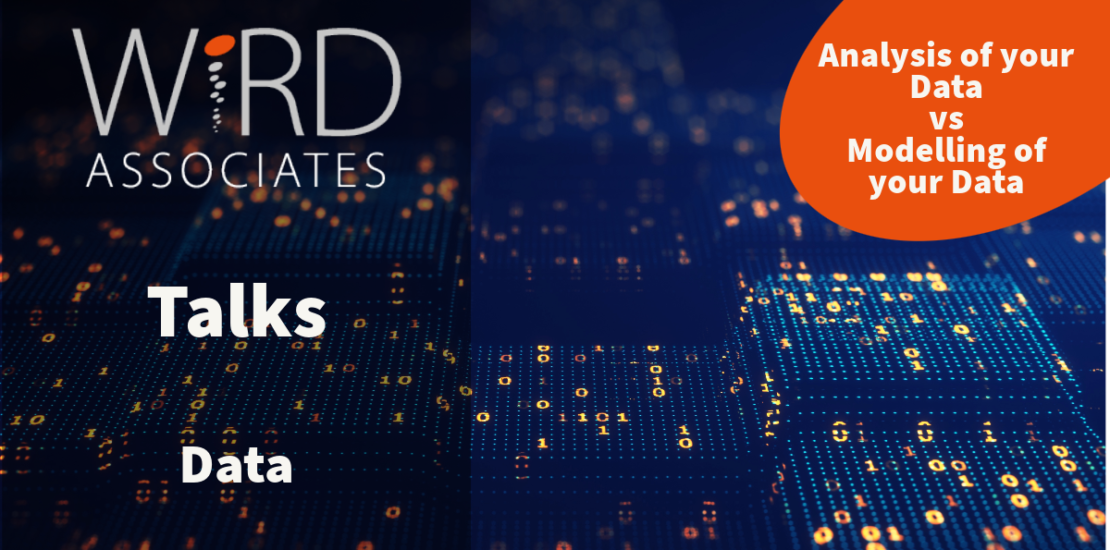
It is not a matter of choice – You’ll need an approach to combine the two for decision-enabling insights.
“Data modelling refers to the architecture that allows data analysis to use data in decision-making processes and data analysis actually puts the model in motion and leverages data to drive outcomes” – www.techrepublic.com
Below are some ways of analysing data:
Statistical Data Analysis: When collecting large volumes of data and using statistics and data analysis techniques to produce identifiable patterns, insights, and trends.
Inferential Data Analysis: Essentially a type of statistical analysis whereby analysing data from smaller data samples of that group generates conclusions about the larger group.
Diagnostic Data Analysis: Understanding of data that focuses on why things happen. It attempts to identify the root causes by first identifying patterns then analysing trends and analysing data between variables that are correlated.
Data mining: Scanning through large data sets to identify relationships and patterns. The goal is finding solutions to specific problems hidden in plain sight simply because of the magnitude. To harvest selected data using patterns. This could prevent problems that are looming based on wisdom gained from previously identified issues.
Predictive Data Analysis: Tools like Data mining can be employed to get data features (specific data) to project (predict) future possible events and likely trends. AI-Technology and Machine-Learning tools can drive predictive analytics that are very complex, and the analysis can then leverage these algorithms to great success.
Prescriptive Data Analysis: A type of Data-Analytics and Data mining that can recommend the best course of action by using and analysing historical data for achieving favourable outcomes.
Data Modelling
The process to represent and store data by creating and using a data model. It is a representation of the related entities involved in aspects of applications, the relationships between those entities and their attributes. Data models are presented in the form of diagrams or tables.
It can be used in both the pre- and post-processing phases of the data analytics process.
It can be used in pre-processing to extract raw data from different sources to build datasets for analysis that are “unified”. Once created, these datasets can enable better combination of insights, insights that are more powerful. As a post-processing technique, it provides enhanced possibly visual detail that stakeholders cannot simply glean alone through descriptive statistics. By using the tools included in data modelling software for advanced visualisation, analysts can display see relationships within their datasets. This is usually impossible to describe but displaying it provides clarity on the information and relationships – Sailing out of the fog.
Analysts use these tools to pivot columns and rows, sort by specific variables, drill down into categories, aggregate data, explore dimensions like projected time or periods of time, locations or geographical features, or filter by keyword search results. These tools simplify building complex codeless queries, extracting, and inserting data into relational databases, generating effective projections without complex calculations, and converting table structures from unstructured data.
The important step toward true data freedom and clarity in your organisation is Data modelling Software because it enables increased visibility on all levels of the analytical process.
To stay relevant, never mind competitive in today’s data climate, Data Modelling software is of crucial importance.
Top Data Modelling tools in 2023
As can be seen from this blog, the combined approach of data analysis and data modelling, if interpreted and analysed efficiently, can help to enable any organisation’s journey to a fully-fledged digital business model that can compete in the current information “Big Bang”.
It will provide decision-enabling analysis of the organisation’s data in ways that used to be impossible before. It can provide calm after the chaos, so to speak.
In WiRD’s experience, many organisations who have invested resources in Data Modelling and Data Analysis, are maybe only using a fraction of the capability of these amazing systems and tools. It can be a very powerful enabler of digital transformation if used in guiding formal enterprise architecture.
What has your experience been in this regard? How effectively is your organisation using its investment in its data? How are the data scientists and other data resources applying themselves to continuously analyse the environment?